Neurocybernetics
„Thanks to neuroanatomy, you can visit the brain, thanks to neurophysiology, you can learn how it works, and neurocybernetics gives you the unique opportunity that, thanks to its methods and models, you can try to understand the brain.” (Ryszard Tadeusiewicz)
Neurocybernetics is a combination of knowledge about biological systems and knowledge about information transfer in humans and machines [1]. In neurocybernetics the inspiration for IT solutions are the anatomical aspects of the nervous systems and the brain. Neurocybernetics integrates computer knowledge with advanced electronic, chemical and semiconductor engineering.
Our lab cooperates with Universidade NOVA de Lisboa. As part of the collaboration, new neuron models are created based on the structure of the thalamus and the cusp catastrophe theory. In our research, we also integrate knowledge in the fields of neural networks and quantum computing.
We use third-generation neural networks, which simultaneously improve the perception of the AI model and the energy efficiency of computing systems. In our laboratories we use various acceleration techniques: GPU, TPU, CMOS, FPGA and Loihi neuroprocessor.
Topics of our projects:
- Spiking Neural Networks (SNN)
- Neuron models
- Supervised/unsupervised learning
- Neuromorphic hardware
- Neural prostheses
- Explainable SNN (XAI SNN)
- Sensor data analysis
- Edge computing with SNN
- Event data processing
- Frameworks and neuroprocessors
- DVS data classification
The laboratory is currently pursuing three doctorates in the field of neurocybernetics.
You can read more about ongoing projects on the SNN team website:
[1] Norbert Wiener (1894 r. – 1964 r.), Cybernetics or Control and Communication in the Animal and the Machine. New York 1948.
Grants
Statutory Activities for Young Staff No. 0311/SBAD/0714
Statutory Activities for Young Staff No. 0311/SBAD/0731
Publications
Overview of Spiking Neural Network Learning Approaches and Their Computational Complexities / Paweł Pietrzak (WIiT), Szymon Szczęsny (WIiT), Damian Huderek (WIiT), Łukasz Przyborowski (WIiT) // Sensors – 2023, vol. 23, iss. 6, s. 3037-1-3037-19
Explainable spiking neural network for real time feature classification / Szymon Szczęsny (WIiT), Damian Huderek (WIiT), Łukasz Przyborowski (WIiT) // Journal of Experimental & Theoretical Artificial Intelligence – 2022, vol. 35, no. 1, s. 77-92
Spiking Neural Network with Linear Computational Complexity for Waveform Analysis in Amperometry / Szymon Szczęsny (WIiT), Damian Huderek (WIiT), Łukasz Przyborowski (WIiT) // Sensors – 2021, vol. 21, no. 9, s. 3276-1-3276-16
60 pW 20 μm size CMOS implementation of an actual soma membrane / Szymon Szczęsny (WIiT), Damian Huderek (WIiT) // Journal of Computational Electronics – 2020, vol. 19, iss. 1, s. 242-252
Spiking Neural Network Based on Cusp Catastrophe Theory / Damian Huderek (WI), Szymon Szczęsny (WI), Raul Rato // Foundations of Computing and Decision Sciences – 2019, vol. 44, no. 3, s. 273-284
A Spiking Neural Network Based on Thalamo-Cortical Neurons for Self-Learning Agent Applications, Damian Huderek, Szymon Szczęsny, Paweł Pietrzak, Raul Rato, Łukasz Przyborowski, vol. 34, Issue 3, pp. 467-483, 2024
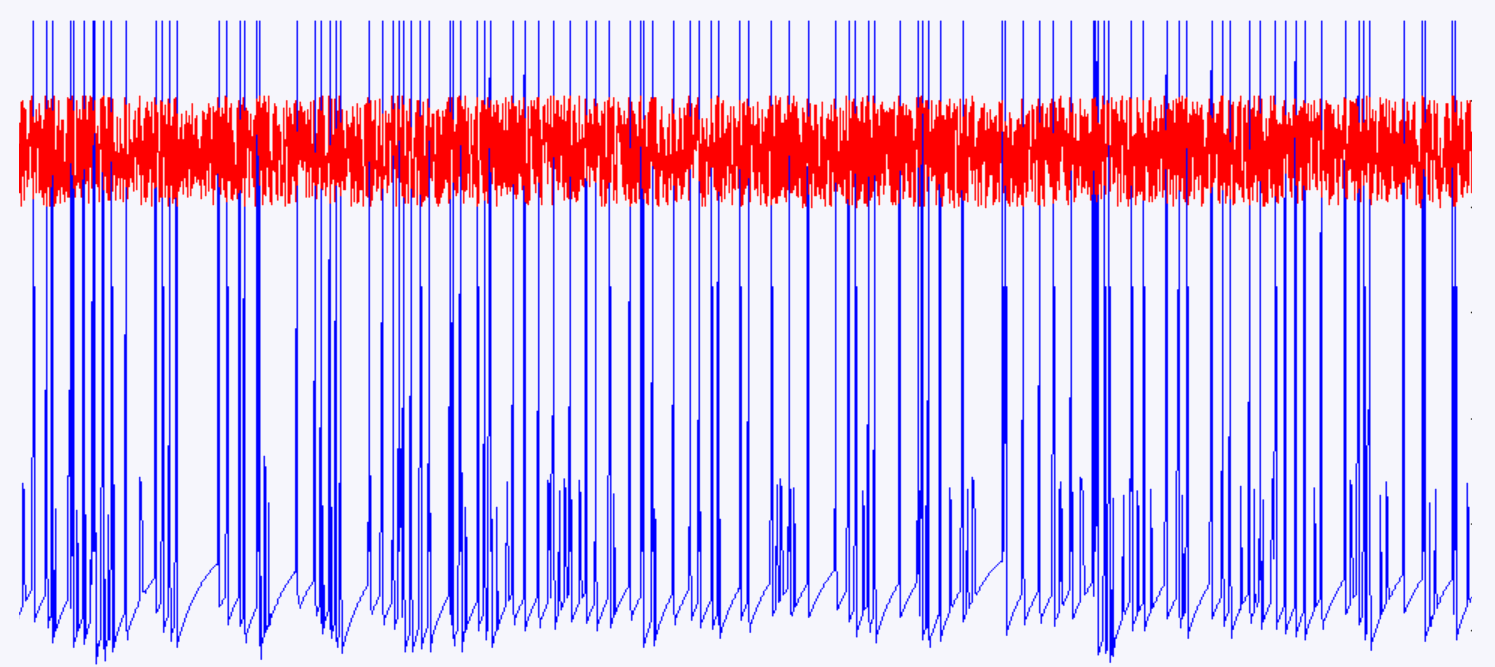
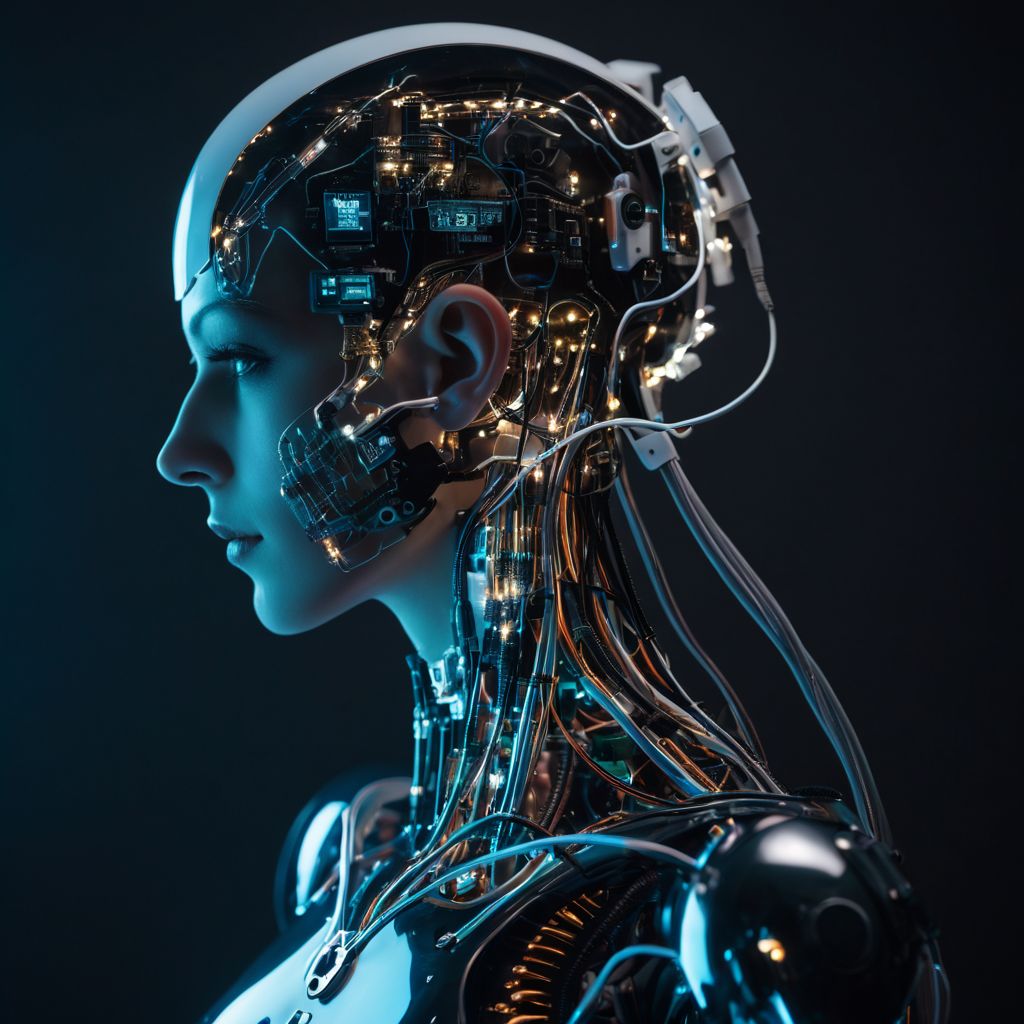